Ping! A Google News notification, “This Coffee Drinking Habit May Help Your Brain,†appears. I don't have time to read it now, but I pour myself an extra cup of coffee, feeling justified. The next day, ping! Another Google News notification: “Too much coffee can cause your brain to shrink, increasing your dementia risk by over 50%.†What? Was I increasing my dementia risk with my extra cup yesterday? Or decreasing it? How can we trust science with contradictory information? Maybe a bit of statistics can help cope with the uncertainty I'm feeling…
While some might have taken a statistics class before leaving high school, most get just enough for our basic science classes, if any. If we really think back, we might remember something about null hypotheses and p-values less than 0.05 being important. These ideas broadly come from one interpretation of statistics, called frequentist statistics. In this interpretation, we define probability as how common something is over a long number of repeated experiments.
Coming back to our coffee example, suppose that we want to know if coffee causes dementia. We collect a random group of patients who either drink coffee or not for 5 years and keep track of how frequently they develop dementia. If many more patients in the coffee-drinker group develop dementia compared to our coffee-abstinent group, we can show that there is an effect on dementia risk from coffee consumption. This is similar to how Kitty Pham and others at the University of South Australia reached their conclusion that drinking large amounts of coffee increases dementia risk. However, one study alone doesn't necessarily mean that we know “the truthâ€!
While there might be scientific criticisms specific to this study, we likely don't have all the expertise to identify those types of issues. However, there is another potential problem in trusting this headline without digging deeper: frequentist statistics assume repeated experiments confirming the results. When studies aren't replicated, results can prove inaccurate. There is a so-called replication crisis in psychology resulting from old studies finally being repeated but finding conflicting results. But if one study alone doesn't show us the truth, how can we make sense of things? I don't have time to thoroughly research and understand the various buttons on my dishwasher, let alone understand neuroscience.
That's where a bit of Bayesian intuition comes in! In contrast to the frequentist statistics which make assumptions around experiment repetition and shun subjectivity, under a Bayesian interpretation of statistics, we express probability as a degree of belief in an event. Usually, this degree of belief is based on prior knowledge, personal beliefs, or conditions related to the event. Bayesian methods then leverage Bayes’ theorem to compute and update probabilities (or degrees of belief) after getting new data. To use an analogy, suppose we're trying to identify a weird beeping sound in our apartment. A frequentist approach might be to wait for the next beep in each room to narrow things down to a particular area, then wait until the beeps get progressively louder and we're able to identify the smoke alarm in question. Someone using a Bayesian approach might recognize the sound as a smoke alarm from their prior knowledge, then check each smoke detector, skipping the one they remembered replacing last week.
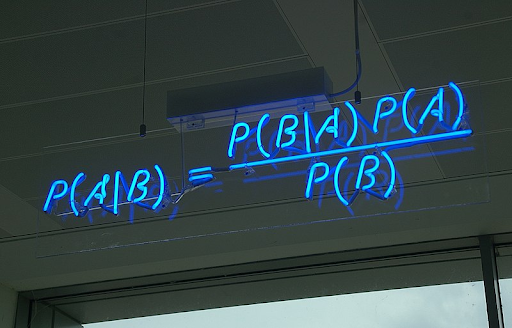
Returning to our coffee example one last time, my prior belief was that while what we eat and drink matter, most things are fine in moderation. Additionally, I know of at least one article showing that coffee decreases your risk of dementia. Now unfortunately, I don't have a group of people willing to skip coffee for the rest of their lives so I can't repeat these two experiments. What I can do is take my existing knowledge and beliefs about coffee, look at the new information from the University of South Australia, and make a decision. I don't know about you, but I'll pour myself another cup until more research shows I need to update my beliefs…
The Bayesian approach can be more intuitive than the frequentist approach, as it allows us to think about statistics as a series of updates to what we know. However, two people with two different sets of prior beliefs may end up at different conclusions with the same data. There is a broader philosophical debate around which is better and in what situations, but that's for another day. More importantly for us, Bayesian statistics can also give us a different philosophical approach to reading science. No one is an expert on every topic, and it's difficult to stay up to date, especially when scientists are still researching questions we take an interest in. While frequentist statistics can sometimes lead us to believe that all science makes clear cut distinctions, like the difference between a black coffee and a flat white, reading science through a Bayesian lens can help us find comfort in the more uncertain areas of scientific research. The next time I encounter new information that seems contradictory, I'll look at the available research, incorporate my prior beliefs and intuition about the topic, and make a decision. While making decisions under uncertainty is uncomfortable, maybe we can take comfort in the fact that scientists are still on the case.
About the Author
Guppy is a third-year bioinformatics Ph.D. student. They have a master's degree in statistics from NC State University, an undergraduate degree in mathematics from Clemson University, and spent a few years working for TIAA as a data scientist before heading to UGA. Their research focuses on studying the evolution and spread of SARS-CoV-2 and highly pathogenic avian influenza using statistical and computational methods. Outside of the lab, Guppy can be found bouldering at Active Climbing, reading science fiction and history, going on hikes with their puppy, Zoya, roasting and drinking coffee, or playing tabletop role-playing games like Dungeons and Dragons.
-
Guppy Stotthttps://athensscienceobserver.com/author/guppy-stott/February 29, 2024
-
Guppy Stotthttps://athensscienceobserver.com/author/guppy-stott/
-
Guppy Stotthttps://athensscienceobserver.com/author/guppy-stott/November 30, 2022